Dr Rajiv Senapati, Assistant Professor, Department of Computer Science and Engineering, along with Dharani Sabari, Ph.D. scholar has published a patent titled “A System And Method For Predicting Network Traffic in Next-Generation Cellular Networks” in the Patent Office Journal. Through their research, they introduced a new model that can predict traffic in networks more accurately while using fewer resources.
Abstract
This study shows a more advanced way to predict traffic in 5G networks. It does this by using a temporal attention mechanism along with a lightweight convolutional layer and hybrid attention layers. The model enhances prediction accuracy by focusing on the most relevant historical data to effectively capture complex traffic patterns. By assigning different weights to key data points, the attention mechanism ensures more precise forecasting. Experimental results indicate that the model outperforms baseline methods, achieving lower Mean Absolute Error (MAE) and Root Mean Square Error (RMSE) across three data services. With fewer parameters and reduced computational demands, the model is highly efficient and suitable for real-time applications. This approach offers a scalable and accurate solution to meet the evolving needs of next-generation cellular networks.
Explanation in Layperson’s Terms
In 5G networks, accurately predicting traffic is crucial to managing resources efficiently and ensuring smooth performance. This is especially important for advanced applications like video calls, virtual reality, and self-driving cars, which require fast response times and reliable connections. By estimating how much data will be used in advance, networks can prevent congestion and maintain consistent service, even during busy times. There are many ways to predict network traffic, but each method has strengths and weaknesses.
Through their research, they introduce a new traffic prediction model that combines two advanced techniques: lightweight convolution and a temporal attention mechanism. Lightweight convolution is a simplified machine-learning method that quickly identifies important patterns in data with minimal processing power. Meanwhile, the temporal attention mechanism captures how network traffic changes over time, identifying key trends and fluctuations. By combining these techniques, the model predicts traffic more accurately while using fewer resources. Tests indicate it outperforms traditional methods by providing more precise predictions with lower errors across three different types of network data. Its low computing power requirements and fewer parameters, make it faster and well-suited for real-time use in 5G networks. Their innovative approach offers a scalable and accurate solution to meet the growing demands of modern, data-heavy applications.
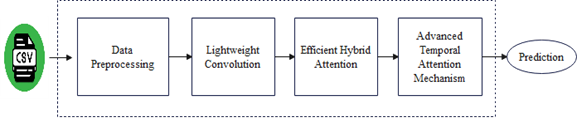
Fig (1) Proposed framework
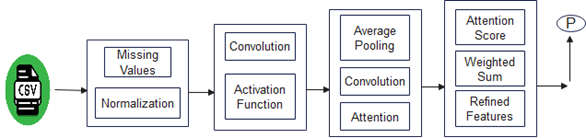
Fig (2) Architecture of the proposed framework
Practical Implementation/Social Implications of the Research
The invention has important applications in smart cities, self-driving vehicles, network resource management, and real-time traffic monitoring. It optimizes traffic flow, improves autonomous car navigation, efficiently manages network capacity, and provides navigation systems with up-to-date traffic data. The advantages include increased quality of service with low latency, energy savings through intelligent resource allocation, scalability to accommodate more connected devices, and better overall user experience with less congestion. These characteristics make the invention an appropriate solution for the rapidly growing 5G network environment, addressing significant challenges in modern traffic and network management.
Future Research Plan
The team will focus on enhancing the model for both 5G and emerging 6G networks by improving traffic prediction accuracy and efficiency. This includes optimising resource allocation and capacity estimation to better manage network demands. Future work will also integrate diverse data sources, such as IoT devices and satellite networks, to improve predictions across different environments. Additionally, efforts will be made to reduce computational costs, enable dynamic traffic adaptation during peak times, and explore advanced AI techniques like federated learning for secure, real-time forecasting. These advancements aim to ensure more reliable and efficient network performance as technology evolves.